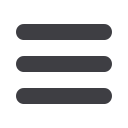
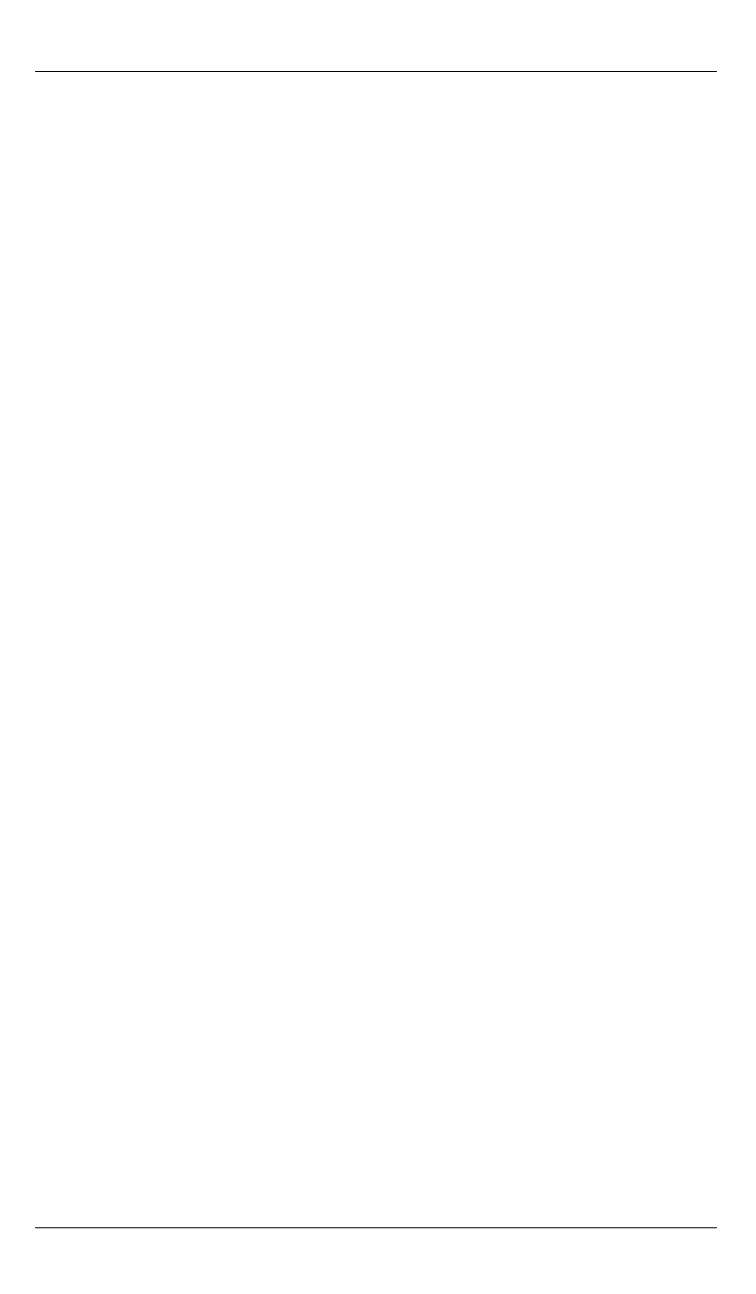
С.Ю. Гуськов, В.В. Лёвин
14
Confidence interval estimation for quality factors of binary
classifiers – ROC curves, AUC for small samples
©
S.Yu. Gus’kov, V.V. Lyovin
JSC “Bank ZENITH”, Moscow, 127566, Russia
Polynomial distribution being presented as conditional joint distribution of independent
Poisson random variables we build confidence intervals for sum polygons based on
grouped data. We then use these estimates to build confidence intervals for ROC curves.
These estimations then could be used in automatic defect detection and quality control
procedures to find and to identify inhomogeneities and anomalies in structure of con-
structional materials and their elements for the end to improve robustness and efficiency
of these procedures for small samples.
Keywords:
confidence intervals, sum polygons, connection between polynomial distribu-
tion and Poisson distribution, ROC curves, binary classifiers
.
REFERENCES
[1]
Engelmann B., Hayden E., Tasche D. Testing rating accuracy.
RISK,
2003,
vol. 16, pp. 82
−
86.
[2]
Stein R.M. Benchmarking default prediction models pitfalls and remedies in
model validation.
J. of Risk Model Validation
, 2007, vol.1, no. 1, pp. 77
−
113.
[3]
Bol’shev L.N.
Teoriya veroyatnostey i ee primenenie — Theory of Probability
and its Applications
, 1962, vol. 7, pp. 353–355.
[4]
Bol’shev L.N.
Teoriya veroyatnostey i ee primenenie — Theory of Probability
and its Applications
, 1965, vol. 7, pp. 356–358.
[5]
Garwood F. Fiducial limits for Poisson distribution.
Biometrica
, 1936, vol. 28,
pp. 437
−
442.
[6]
Stevens W.L. Fiducial limits of the parameter of discontinuous distribution.
Biometrica
, 1950, vol. 37, pp. 117
−
129.
[7]
Sofus A. Macskassy and Foster Provost, Confidence Bands for ROC Curves:
Methods and an Empirical Study.
CeDER Working Paper 02
−
04.
Stern School
of Business, New York University, Jan. 2004, 15 p.
[8]
Jokiel-Rokita A., Pulit M. Nonparametric estimation of the ROC curve based
on smoothed empirical distribution functions.
Statistical Computing,
2013,
vol. 23, pp. 703–712.
[9]
Baklizi A. A Simple Method for Finding Emperical Liklihood Type Intervals for the
ROC Curve.
J. of Modern Applied Statistical Methods
, 2007, vol. 6, no. 2,
pp. 589
−
595.
[10]
Le Meur Y., Vignolle J.-M., Chanussot J. Practical use of receiver operating
characteristic analysis to assess the performances of defect detection algorithms.
J. of Electronic Imaging, Society of Photo-optical Instrumentation Engineers (SPIE)
,
2008, vol. 17, no. 3, pp.10.1117.
[11]
Dobrzański L.A., Krupiński M., Sokolowski J.H. Methodology of automatic quality
control of aluminium castings.
J. of Achievements in Materials and Manufacturing
Engineering
, 2007, vol. 20, no. 1–2, pp. 69–78.